AI Safety in China #5
China-US intergovernmental dialogue on AI, provincial policies on frontier AI, Shanghai AI Lab alignment work, and opportunities at Concordia AI
Key Takeaways
China and the US announced the creation of an intergovernmental dialogue on AI, which could include the “risks of advanced AI systems” and improving “AI safety.”
Shanghai and Guangdong announced policies on large models and AGI respectively, including provisions on model testing and evaluation, as well as early warning of AGI risks.
Shanghai AI Lab-led teams published preprints on LLM alignment, including the first Chinese LLM alignment benchmark incorporating traditional Chinese principles of morality.
The Shanghai AI Industry Association held a forum on AI safety and alignment together with the Shanghai Xuhui district government.
International AI Governance
China and US announce new governmental dialogue on AI
Background: On November 15, Chinese President Xi Jinping and US President Joe Biden held a bilateral meeting during the Asia-Pacific Economic Cooperation (APEC) summit in San Francisco.
Discussion of AI: Both the Chinese and US government readouts noted discussion on AI. According to the Chinese government, both countries will strengthen cooperation on AI, including creating an intergovernmental dialogue on AI. Meanwhile, the US noted under areas of progress that “the leaders affirmed the need to address the risks of advanced AI systems and improve AI safety through U.S.-China government talks.” AI was listed as the first area of cooperation in the Chinese readout, compared to third in the US readout, which may indicate relative prioritization of the issue.
Implications: It is currently unclear when talks will occur, what the dialogue mechanism will be, and what topics would be covered. The US readout’s mention of “advanced AI systems” suggests there may be focus on frontier AI safety. We believe that productive areas of conversation between the US and China on AI safety could include: which standards should be enacted at international versus national levels, increasing the proportion of government and industry funding for AI safety and governance research compared to capabilities research, and sharing lessons learned in governing AI domestically. Bilateral China-US discussions are crucial to any international consensus on frontier AI governance and important to reducing AI capabilities racing dynamics.
Domestic AI Governance
Two provincial-level jurisdictions release policies regarding frontier AI
Background: On November 7, Shanghai released a policy on large model innovation (2023-2025).1 This was followed the following week by a Guangdong province policy on artificial general intelligence (AGI) innovation. Other jurisdictions with similar policies include Beijing and Chengdu.2
Similarities and differences in policies: Both policies primarily focus on development and innovation, including improving computing supply, building and sharing data corpuses, and promoting applications. The Guangdong policy, which is more lengthy and detailed, also focuses on expanding the role of the Guangdong–Hong Kong–Macao Greater Bay Area. Both policies discuss testing and evaluation, with the Shanghai policy specifically calling for creating a national-level large model testing and evaluation center to evaluate model capabilities, safety/security, ethics, and compatibility. The Guangdong policy has several other safety-related policies absent in Shanghai. For example, it notes support for increasing AI security and promoting watermarking technology. It also discusses improving AI oversight through a tiered supervision mechanism, broken up into high, medium, and low-risk applications. Additionally, it calls for conducting early warning of risks and catastrophes from AGI, instituting safety/security norms, researching ethics, and pursuing compatibility with international standards.
Implications: China has a long tradition of trialing policies at the local level before instituting them nationally, and Shanghai and Guangdong are the two most important frontier AI hubs in China outside of Beijing, so these policies foreshadow possible national-level policies. Given the creation of AI Safety Institutes in the UK and US for tasks including testing of frontier AI models, the creation of a Shanghai large model testing and evaluation center could be a precursor to developing a national institution in China. The safety-conscious provisions in these policies suggest that some government efforts are being devoted to preventing frontier AI risks, though development remains the main emphasis.
Technical Safety Developments
Shanghai AI Lab and Fudan release new papers on alignment
Background: Shanghai Artificial Intelligence Laboratory (SHLAB) and Fudan University collaborated on two new preprints in November regarding large language model (LLM) alignment. The creation of SHLAB was announced at the 2020 World AI Conference (WAIC). The group that published the first preprint was led by WANG Yingchun (王迎春), deputy head of the SHLAB Governance Research Center, and QIAO Yu (乔宇), assistant to the director of SHLAB. The second preprint was led by Wang Yingchun as well as LIN Dahua (林达华), associate professor at the Chinese University of Hong Kong (CUHK), Director of the CUHK-SenseTime Joint Laboratory, and affiliated with SHLAB, as well as Fudan Natural Language Processing Group’s QIU Xipeng (邱锡鹏).
“Fake Alignment” paper: The first preprint displayed preliminary results showing that LLMs are much better at answering open-ended questions than multiple-choice questions on safety evaluation datasets, which they argue is caused by mismatched generalization. They termed this phenomenon “fake alignment,” since models are remembering answers to safety questions rather than truly “understanding” safety. Inspired by research on jailbreak attack patterns, they construct a Fake alIgNment Evaluation (FINE) framework that measures degree of consistency between multiple-choice and open-ended questions, testing it across 14 models using categories of fairness, individual harm, legality, privacy, and social ethics. This attempts to show limitations with existing evaluation methods.
FLAMES benchmark: The second paper creates a benchmark named FLAMES to evaluate value alignment of Chinese LLMs. The authors seek to improve upon existing Chinese benchmarks by providing “highly detailed” annotation guidelines and a specified scoring model trained on labeled data to evaluate responses to FLAMES prompts. The evaluation encompasses five dimensions of human values: fairness, safety, morality, data protection, and legality; the morality dimension includes Chinese cultural and traditional qualities such as harmony, benevolence, and courtesy. Evaluating on 12 models, they find that Claude is the best-performing, but i still scores only 63%, indicating significant gaps for LLMs on value alignment.
Implications: These two papers highlight that serious work on alignment is coming out of SHLAB. The first paper is interesting as it showed the large discrepancy in the performance between multiple-choice questions and open-ended questions in safety test datasets. The second paper is the first Chinese LLM alignment benchmark we are aware of to incorporate traditional Chinese principles of morality, adding to the global debate around which human values we should align LLMs to.
Researchers use LLMs to improve interpretability
Background: A research team led by Microsoft Research Asia (MSRA) Senior Principal Research Manager and Societal AI team lead XIE Xing (谢幸) and University of Science and Technology of China School of Computer Science and Technology Vice Dean LIAN Defu (连德富) published a preprint titled “RecExplainer: Aligning Large Language Models for Recommendation Model Interpretability.”
Paper content: The paper seeks to use LLMs as a surrogate model to assist in interpretability of recommender systems. They use three approaches to achieve this goal: behavior alignment, where LLMs are trained to emulate the recommender model’s patterns, intention alignment, wherein the LLM is trained to understand embeddings (activations of neural layers) of the recommender system, and hybrid alignment, which mixes the two approaches. They then test their model on three datasets—Amazon Video Games, Amazon Movies & TV, and Steam. The authors argue that their procedure is effective at both comprehending and explaining recommender algorithms.
Implications: The conclusions of this paper seem to offer a new, AI-powered, path to interpretability for standard AI recommendation models.
Other relevant technical publications
University of Science and Technology of China and Beijing University of Posts and Telecommunications MOE Key Laboratory of Trustworthy Distributed Computing and Service, On the Calibration of Large Language Models and Alignment, arXiv preprint to be published in findings of EMNLP-2023, November 22, 2023.
Expert views on AI Risks
Shanghai association holds seminar on AI safety and alignment
Background: On November 19, a forum on “Large Model Safety and Alignment” was held by the Shanghai AI Industry Association (上海市人工智能行业协会) (SAIA). SAIA safety and security director CHEN Xi (陈曦) led the event under the guidance of Shanghai Xuhui District Science and Technology Commission deputy director YU Wei (虞蔚). Other notable attendees include a senior researcher from Tencent Research Institute, a deputy director of the Shanghai Key Laboratory of Computer Software Testing and Evaluating and a new Generative AI Quality Testing Center, as well as professor LI Bo (李博) from the University of Chicago and University of Illinois at Urbana-Champaign (UIUC). 30 other private companies, government-affiliated labs, and universities also attended.
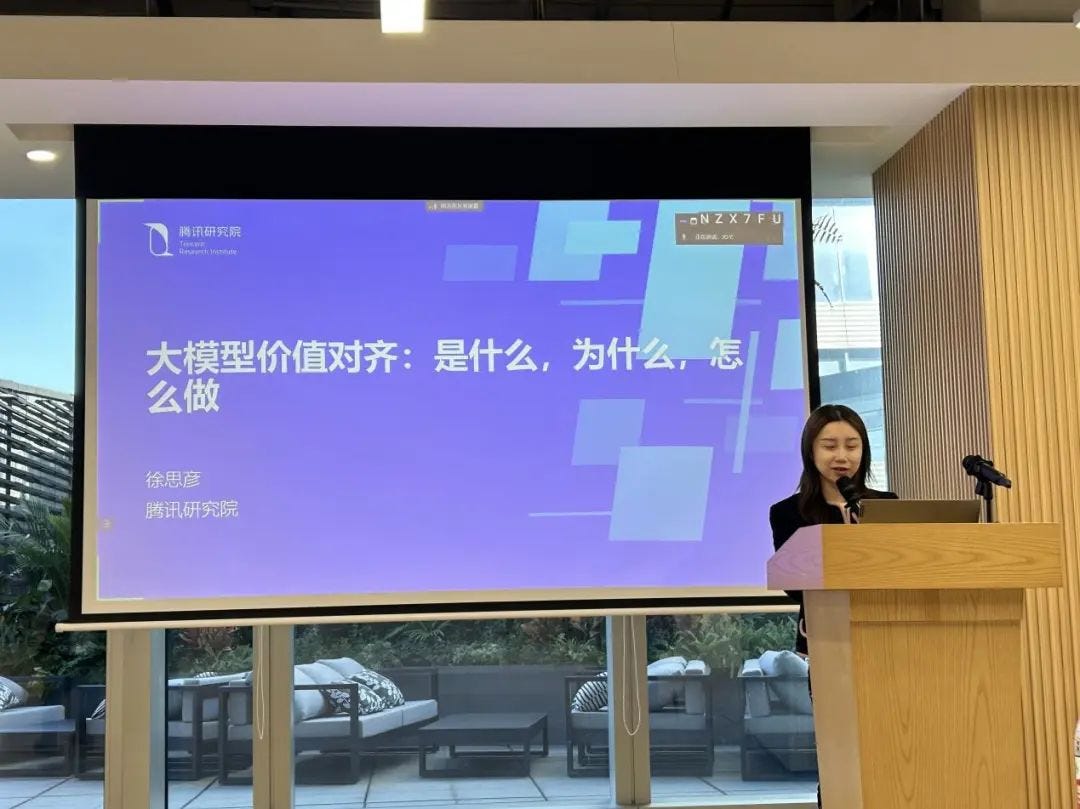
The forum: The event included presentations on AI safety and security issues, including the necessity of achieving value alignment, relevant standardization work on large models, evaluating LLMs for hallucinations and robustness, quantitative evaluations for large models, and assessing trustworthiness and risks of LLMs. Participants also discussed similarities and differences between English and Chinese benchmarks on AI safety and alignment.
Implications: This appears to be the first public event held by SAIA on AI safety and alignment. The inclusion of a Shanghai local government official and various figures in industry and academia show the growing interest in AI safety issues in China. Shanghai could become an important pilot area for AI testing and evaluation, given its recent policy on large AI models, and both industry and academia will likely play an important role in shaping this project.
Chinese scientist discusses frontier AI risks in party newspaper
Background: On November 15, AI scientist GAO Wen (高文) published an op-ed about transformations brought about by AI in the Study Times (学习时报). Gao Wen is director of Shenzhen-based research lab Peng Cheng Laboratory, Dean of the School of Information Science and Technology at Peking University, and an academician of the Chinese Academy of Engineering. In previous work translated by Concordia AI, he expressed concerns about AGI risks and discussed the importance of international cooperation. The Study Times is the official newspaper of the Central Party School, an institution for training party cadres.
His op-ed: In the article, Gao Wen explained the history of AI development, discussed paths to “strong” AI, outlined China’s advantages and gaps in AI, and suggested paths forward. In discussing AI risks, he noted the importance of taking precautionary steps to prevent loss of control of AI so that humans are not enslaved and manipulated by AI. He argued that it is important to constrain AI from the legal and moral levels. He had six main recommendations: elevating AI’s importance to a national development strategy, improving the R&D system, better developing talent, strengthening AI infrastructure, accelerating research on laws and ethics regarding AI to ensure safety and controllability, and deepening international cooperation and participating actively in global governance.
Implications: Given Gao Wen’s role as a senior scientist and policy advisor, including presenting at a Politburo study session on AI in 2018, his continued remarks about frontier AI risks could further influence government policies to address these concerns. It is particularly interesting that this article was written for a party cadre audience in the Study Times, rather than a scientific audience.
What else we’re reading
Sundar Pichai and Emily Chang, Google CEO on China vs US AI Race, Bloomberg Television, November 17, 2023.
Will Henshall and Anna Gordon, Why China’s Involvement in the U.K. AI Safety Summit Was So Significant, Time, November 3, 2023.
Mustafa Suleyman, Mariano-Florentino (Tino) Cuéllar, Ian Bremmer, Jason Matheny, Philip Zelikow, Eric Schmidt, Dario Amodei, Proposal for an International Panel on Artificial Intelligence (AI) Safety (IPAIS): Summary, October 27, 2023.
Opportunities at Concordia AI
Concordia AI is hiring for full-time and part-time staff
Concordia AI is hiring for two full-time roles: Technical Community Manager and Governance Researcher.
We are also hiring part-time affiliates to join one of four working groups: AI safety technical content, AI safety outreach and strategy, Chinese AI governance, and International AI governance.
To learn more about the positions, please see this link. You can apply using this form. All positions require proficiency in Mandarin.
We are also organizing an online Q&A session for hiring, which you can RSVP for here.
Feedback and Suggestions
Please reach out to us at info@concordia-ai.com if you have any feedback, comments, or suggestions for topics for the newsletter to cover.
Shanghai is one of four “directly-administered municipalities” (直辖市), which means that it has the same administrative level as a province.
See pages 19-21 of our State of AI Safety in China report.