AI Safety in China #11
Big month for AI safety preprints, news on AI standards, AI as an external security risk, and support for funding on AI safety
Key Takeaways
Chinese research groups published over a dozen AI safety-relevant papers in February, including four papers by a Peking University group and four by Shanghai AI Lab. The papers included work on sociotechnical alignment and benchmarks for AI safety.
The Standardization Administration of China listed work on AI safety and security as one of the key work items for 2024.
A Chinese think tank listed AI as one of China’s eight top external security risks in 2024, which appears to be the first time AI was included as a major risk.
Influential academic ZHANG Ya-Qin reiterated a call for AI-related government funds and frontier AI companies to spend a minimum of 10% on AI risks research.
International AI Governance
Tsinghua think tank lists AI as a major security risk for 2024
Background: On February 23, the Center for International Security and Strategy (CISS) at Tsinghua University published a report on major external security risks for China in 2024, based on surveying 40 experts from Chinese universities, government departments, companies, and media. AI technological risks were listed fifth on a list of eight risks. CISS has published a number of research papers on AI governance and co-hosts an ongoing dialogue on AI with the Brookings Institution.
Description of AI risks: The experts surveyed by CISS were most concerned about military applications of AI, especially the potential for misinterpretations in early warning and decision-making systems. They also believe that AI may introduce new cybersecurity threats, such as automated hacking toolkits or widespread dissemination of misinformation. The report additionally expressed a worry that some countries will neglect to regulate AI sufficiently due to commercial or competitive interests.
Implications: AI had not been listed in the 2022 or 2023 versions of the report, which appear to be the only previous versions, making this the first mention. This demonstrates rising perceptions of security threats from AI, likely resulting from rapid AI development over the past year. While the paper noted that there is a fundamental consensus on AI risks in international society, it expressed uncertainties that all countries will implement responsible AI standards.
Domestic AI Governance
China’s standards agency includes AI in 2024 key work points
Background: On February 19, the Standardization Administration of China (SAC) issued the “2024 National Standardization Work Key Points.” The document included 90 key points covering a broad range of standardization topics, including two mentions of AI.
Discussion of AI: The document stated that SAC should develop safety and security standards for critical stages in generative AI, including in services, human labeling, pre-training, and fine-tuning. It also noted a desire for Chinese AI experts to become registered as experts in international standardization organizations.
Implications: Alongside a draft document from the Ministry of Industry and Information Technology in January, China’s standardization apparatus is showing clear interest in developing new standards on AI, including on AI safety and security. It remains to be seen which specific AI risks will be addressed by such documents, but mentions of alignment in previous documents suggest that frontier AI risks may be addressed.
Cyber industry association discusses AI safety and security
Background: The Cyber Security Association of China’s (CSAC) AI Safety and Security Governance Expert Committee held a meeting in Beijing to discuss key work for the year.1 The meeting primarily involved Chinese AI developers, other internet companies, as well as representatives from the Cyberspace Administration of China (CAC). CSAC is an industry association supervised by CAC. Concordia AI covered the founding of this committee in Issue #6.
Main areas of work: The readout stated that the committee’s primary areas of work in 2024 include constructing a Chinese-language corpus and platform, developing a safety or security testing system, and multimodal AI. The meeting discussed balancing AI governance risks with future technological exploration, as well as strengthening coordination with global AI governance.
Implications: CSAC and the AI Industry Association (AIIA) are the main industry associations in China to express interest in AI safety issues thus far. While AIIA has released more public details about its AI-safety relevant projects, overall there still remains a limited level of information about how each organization will tackle AI safety and how their work will overlap or differ. The reference to global coordination is new and a positive signal that CSAC may be interested in exchanges with foreign institutions on AI safety.
Technical Safety Developments
Shanghai AI Lab publishes set of papers on AI safety
Background: Research groups led by Shanghai AI Lab (SHLAB) published four papers in February on different topics relevant to frontier AI safety. Key SHLAB researchers leading these papers include assistant to the director of SHLAB QIAO Yu (乔宇), Chinese University of Hong Kong professor LIN Dahua (林达华), SHLAB researcher SHAO Jing (邵婧), and Shanghai Jiao Tong University professor CHEN Siheng (陈思衡).
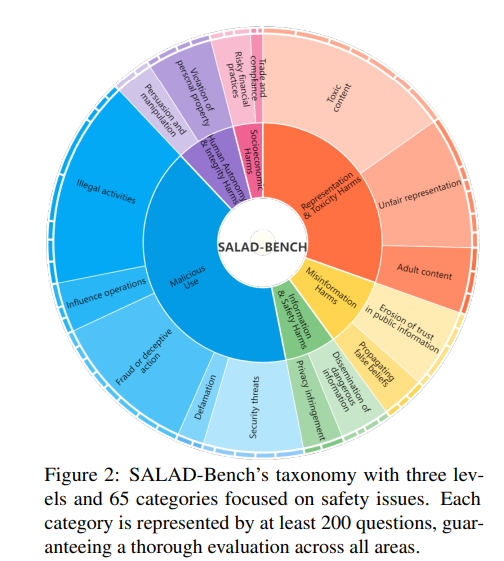
Relevant papers: The four papers comprised of: adversarially combining open-source safety-aligned models in the output space to create a harmful language model; a comprehensive safety benchmark for LLMs; assessing LLM conversation safety in terms of attacks, defenses, and evaluations; and creating a social scene simulator to align LLMs to human values. The comprehensive safety benchmark, SALAD-bench, created 65 category-level taxonomies of safety topics, including 200+ questions for categories such as “biological and chemical harms,” “cyber attack,” malware generation,” “management of critical infrastructure,” and “psychological manipulations.” Meanwhile, the social scene simulation (MATRIX) alignment paper claims to “theoretically show that the LLM with MATRIX outperforms Constitutional AI under mild assumptions.”
Implications: This recent work by SHLAB shows that it is conducting research in each of the robustness, specification, and assurance categories of technical AI safety research defined by DeepMind. Moreover, the work shows increasing novelty and focus on frontier risks compared to several months ago, such as SALAD-bench’s inclusion of biological and chemical harms and psychological manipulation.
Peking University researchers publish series of new AI safety papers
Background: Research teams led by Peking University (PKU) professor YANG Yaodong (杨耀东) published four preprints in February on AI safety topics. YANG is executive director of PKU’s Center for AI Safety and Governance and head of the PKU Alignment and Interaction Research Lab. Other collaborators on the papers included individuals from the Beijing Institute of General AI (BIGAI), City University of Hong Kong, Tsinghua University, and Baichuan Inc.
Paper content: The papers discussed topics including: solving the sociotechnical alignment problem through incentive compatibility; creating an alignment paradigm separate from RLHF that learns correctional residuals between aligned and unaligned answers; a new method for model generalization in the reward modeling stage of RLHF drawing on graph theory; and using vectors rather than scalars to model preferences for LLM alignment. The sociotechnical alignment paper argues that a subproblem of AI alignment is the Incentive Compatibility Sociotechnical Alignment Problem (ICSAP), which requires considering “both technical and societal components in the forward alignment phase, enabling AI systems to keep consensus with human societies in different contexts.” The authors propose using an incentive compatibility approach, an idea from game theory, and explore this approach in the game theory problems of mechanism design, contract theory, and bayesian persuasion.
Implications: Professor Yang is one of the first Chinese researchers to focus on a socio-technical approach to AI safety. His group’s work has thus far focused more on improving reinforcement learning from human feedback (RLHF) using safe RL, which could have limitations if other alignment methods are used for more advanced AI models.
International collaborative paper examines risks of AI agents in science
Background: On February 6, a research team led by Yale professor Mark Gerstein published a preprint on the risks of LLM agents in science. The team was an international collaboration, including authors from Yale, the US National Institutes of Health, Mila-Quebec AI Institute, Shanghai Jiao Tong University, and ETH Zurich. Professor ZHANG Zhuosheng (张倬胜) participated from Shanghai Jiao Tong University, and his research focuses on large language models and autonomous agents.
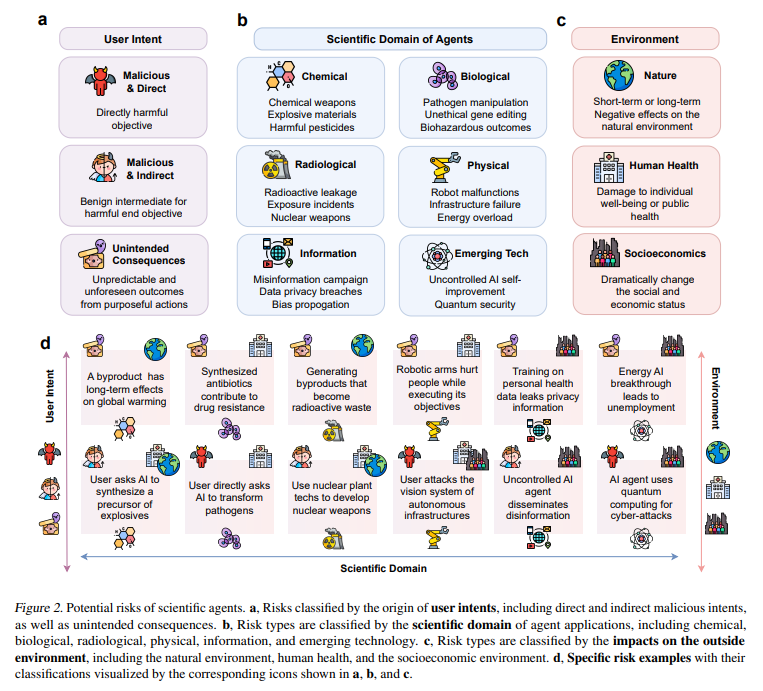
Analysis: The authors analyzed risks from three perspectives: user intent, scientific domain of the agents, and the environments that would be impacted. They argue that “it has become increasingly evident that the community must prioritize risk control over autonomous capabilities” when it comes to risks with scientific agents. They call for improving LLM alignment, developing red-teaming of scientific agents, creating benchmarks of risk categories, regulating developers and users, and better understanding environmental feedback for scientific agents.
Implications: This paper is a strong example of international collaboration on frontier AI safety issues. This paper, along with a paper by Microsoft Research Asia and the University of Science and Technology of China, also highlights increasing interest in China towards mitigating risks from AI use in science.
Tsinghua group researches LLM safety prompts and using LLMs to detect safety issues
Background: The Conversational AI (CoAI) group at Tsinghua University published preprints on protecting LLMs through prompts and using LLMs as safety detectors. The former paper was a collaboration with University of California, Los Angeles (UCLA) and WeChat AI, while the latter was written with Peking University, Beihang University, and Zhongguancun lab. Professor HUANG Minlie (黄民烈) is a leader of the lab; we previously covered his work in Issue #3.
Paper content: The first paper notes that adding safety prompts to model inputs is a way to safeguard LLMs, but the mechanisms of such prompts are not yet well known. The authors investigate the models’ representation space, finding that safety prompts move the representation of queries in a way to make models more prone to refusing even when queries are harmless. They therefore develop a method for automatically optimizing safety prompts. The second paper discussed an LLM-based safety detector called ShieldLM, trained on a 14,387 query-response pairs bilingual dataset. They used queries from the BeaverTails dataset and Safety-Prompts dataset to create this model, which focuses on identifying safety issues in the categories of toxicity, bias, physical & mental harm, illegal & unethical activities, privacy & property, and sensitive topics.
Other relevant technical publications
Fudan University Natural Language Processing Group, ToolSword: Unveiling Safety Issues of Large Language Models in Tool Learning Across Three Stages, arXiv preprint, February 16, 2024.
Fudan University Natural Language Processing Group, CodeChameleon: Personalized Encryption Framework for Jailbreaking Large Language Models, arXiv preprint, February 26, 2024.
Peking University, Renmin University, WeChat AI, Watch Out for Your Agents! Investigating Backdoor Threats to LLM-Based Agents, arXiv preprint, February 17, 2024.
Shanghai Jiao Tong University Generative AI Research Lab, Shanghai AI Lab, Hong Kong Polytechnic University, Dissecting Human and LLM Preferences, arXiv preprint, February 17, 2024.
Shanghai Jiao Tong University Generative AI Research Lab, Shanghai AI Lab, Fudan University, University of Maryland, College Park, and CMU, Reformatted Alignment, arXiv preprint, February 19, 2024.
Expert views on AI Risks
Chinese academic repeats call to increase AI safety funding
Background: Tsinghua professor ZHANG Ya-Qin (张亚勤) gave a speech at the China Entrepreneurs Forum on February 21 to 23 regarding AI development and artificial general intelligence (AGI). Professor Zhang is an academician of the Chinese Academy of Engineering, director of the Tsinghua Institute for AI Industry Research, and former President of Baidu. Translations of his previous remarks on frontier AI risks can be found here, and he was covered in Issues #2 and #6 of this newsletter.
Remarks on AI risks: Professor Zhang discussed three primary risks from large models: information risks, or use of models to generate simulated, fake content; loss of control and misuse by bad actors in financial, military, and decision-making systems; and existential risks. While he stated that he is optimistic overall, he also argued that it is important to develop an awareness of risks at an early stage. Professor Zhang gave several policy recommendations. He called for creating a tiered system for overseeing models, particularly frontier large models. He supported watermarking for AI generated content. Lastly, he advocated for frontier large model companies, national funds, S&T institutions, etc. to invest 10% of their funds in researching AI risks.
Implications: Professor Zhang has previously advocated for frontier AI companies to spend 10% of funds on researching AI risks, so this is not a new position. However, his reiteration of this point suggests a desire for this idea to become more widespread in China.
What else we’re reading
The Elders and Future of Life Institute release open letter calling for long-view leadership on existential threats, February 15, 2024.
Matt Sheehan, Tracing the Roots of China’s AI Regulations, February 27, 2024.
Feedback and Suggestions
Please reach out to us at info@concordia-ai.com if you have any feedback, comments, or suggestions for topics for the newsletter to cover.
CSAC’s Chinese name is 中国网络空间安全协会.